Denoising
Denoising is the process of removing artifacts from raws. This is only applicable to digital raws. There are several different types of artifacting that can occur, and each have their own ways of correcting the artifacting. It is also important to note that all artifact correction removes information from the raws, and thus correction should only be done if the artifacting is egregious, and only the most minimal application of correction should be applied as to not unnecessarily destroy detail.
Identification
The first step to artifact correction is identifying the type of artifact(s) the image(s) have.
Blocking/Quantization/JPEG
This is the most common artifact seen in scanlation
Blocking artifacts, also known as quantization artifacts or JPEG artifacts, are image artifacts that occur due to the way many different compression formats split an image into discrete pixel blocks, and process each block separately. The JPEG format splits an image into 8x8 pixel blocks, then computes the discrete cosine transform of the block. This is a Fourier transform-like transformation, representing the image in the frequency domain. Then depending on the quality setting, the resulting frequency data is then divided by a matrix of constants. The higher frequency data is reduced more than lower frequency data, then the end result is rounded. This often results in a large amount of zeroes or very lower integer values in the high frequency data, which compresses easier.
The blocking artifacts occur when decoding the resultant quantized data. It is the result of one 8x8 block of pixel not smoothly transitioning to the neighboring block of pixels.
Both the easiest and highest quality (destroys the least non-artifact data) technique is to use a neural network trained for reversing this artifact.
- Download https://github.com/chaiNNer-org/chaiNNer
- Download https://openmodeldb.info/models/1x-JPG-20-40, https://openmodeldb.info/models/1x-JPG-40-60, https://openmodeldb.info/models/1x-JPG-60-80, and https://openmodeldb.info/models/1x-JPG-80-100
- These are models the correspond to the JPEG quality the image was saved with, with 80-100 meaning the image was 80% to 100% quality, and therefore it is the weakest model.
The first is a program for applying the neural network filter. The second are model that are good at removing JPEG artifacts, but also preserving other details. Most models are bad at one or both of these, while these are not.
Next a filter plan must be put together. This is fairly straight-forward. Batch load the images, load the model, apply the model, then save the resultant image as PNG.
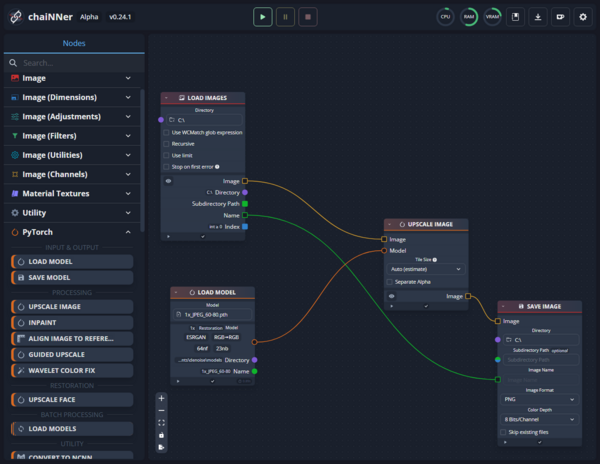
Set the directories to the input and output directories desired. Next the appropriate model must be selected. The easiest way to do this is to select a "representative" page. This page should have straight lines, text, gradients, and screentone patterns. Run only that page through the filter chain with the weakest model (80-100).
Compare the input with the output. Realize that most programs will resample the images in some way, so the way to compare is with a tool that will explicitly show the scale of the images being compared. They must be compared with a nearest neighbor or billinear resampling filter, or at 100% scaling with 1 monitor pixel corresponding to one image pixel. The easiest way to do this is with an internet browser.
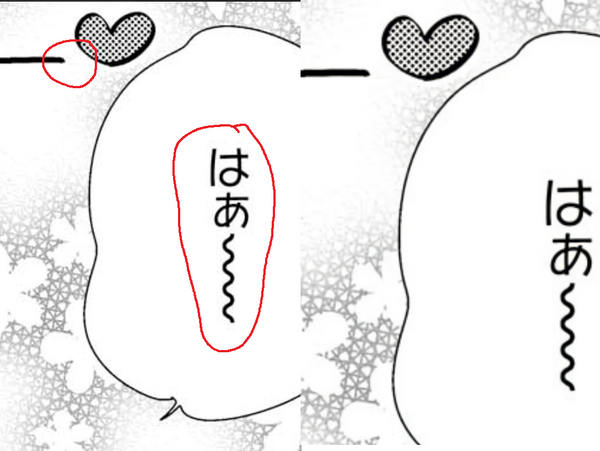
If the artifacts are sufficiently removed, and the rest of the page detail is preserved, then use that model for the rest of the pages. If the artifacting remains, then move to the next strongest and compare again. If too much of the page detail is lost, then move to a weaker model, or if at the weakest already then consider not denoising.
Banding
TODO
Aliasing
TODO
Ringing
TODO